10 min to read
A Layperson's Guide to Managing AI Risks for Businesses
Demystifying the Major Generative AI Risks for Enterprise Leaders
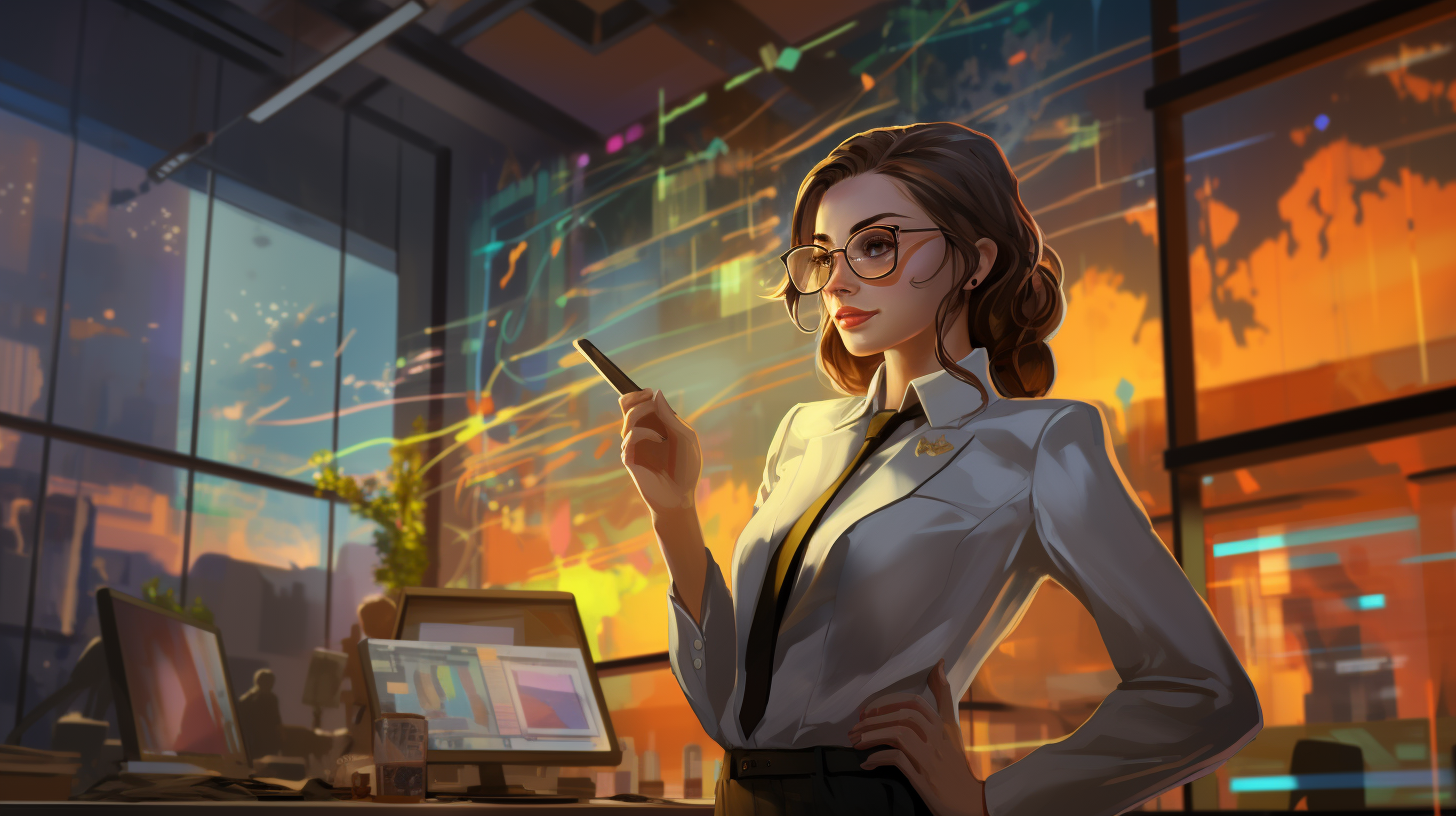
ChatGPT and similar AI tools are causing lots of excitement lately. They can write, code, draw and more! But as with any new technology, they also come with risks for companies.
In this article, we’ll outline the key challenges with AI like ChatGPT in plain English. We’ll also share some smart ways your business can address them.
How Generative AI is Changing the Game
Generative AI systems can make completely new content on their own. Unlike older AI, they don’t just analyze data that humans give them. For example, ChatGPT can write articles, poems, code and more from scratch!
These technologies are spreading incredibly fast. ChatGPT hit 100 million users in just two months! Industry experts say 60% of companies will use AI like this by 2024.
So in many industries, AI will soon become vital to how work gets done. It can handle tasks like:
- Answering customer questions
- Writing marketing content
- Coding software projects
- Analyzing data to spot trends
This will bring big changes to how companies operate. AI can automate manual processes to cut costs. But it also disrupts existing ways of doing things. Companies will need to upgrade skills and security for the new AI-powered era.
“By 2022, 25% of digital workers will use AI daily, up from less than 2% in 2017.” - Gartner
The rapid growth means businesses should start addressing risks now before deploying AI widely. With smart strategies, companies can integrate AI safely and legally.
The Risk of Fake Content
A big appeal of ChatGPT is its amazingly human-like content. But for business uses, false information can be very risky.
AI still makes many mistakes. Without oversight, it could give wrong data or advice to customers. This erodes trust and may cause legal issues.
AI also reflects societal biases. It can generate offensive or harmful content that damages reputations.
Companies must check all AI outputs before use. Review processes to verify facts are essential. AI disclaimers on generated materials are vital too. This minimizes risks to reputation, ethics and legal compliance.
Here’s a specific example:
A bank wants to use AI to generate mortgage advice for customers on its website. But without checks in place, the AI could provide inaccurate financial guidance that seems legitimate. This exposes the bank to legal liability and loss of trust.
By establishing human review of all AI-created mortgage content before publication, the bank ensures advice given is fact-checked. This protects customers and the bank’s reputation.
Securing Sensitive Data
Most AI needs massive data sets to train on. So companies must be very careful giving it access.
Flaws could let hackers steal training data. They could also corrupt the AI by poisoning data. Even authorized access creates risks of intellectual property theft and legal violations.
To secure data, companies need strong access controls, encryption and auditing. They should limit data types shared, enable opt-outs, and restrict permissions. Anonymizing data helps lower risks.
Some vendors claim to anonymize user data. But companies should still demand transparency and audit rights. Handling personal data also requires navigating complex global privacy laws. Hiring qualified legal help is strongly advised.
Here’s an example:
A healthcare provider wants to use AI for research. This involves sensitive patient data. If compromised, the provider could face major HIPAA fines or lawsuits.
By anonymizing data before allowing the AI access, the risk of exposure is reduced. The provider also undergoes strict audits of the AI vendor’s security controls and protocols before deployment. This helps ensure compliance and responsible data handling.
AI-Enhanced Cyber Threats
Unfortunately, AI also gives bad actors new powers. Advanced phishing attacks could use AI-generated personalization and credibility to better exploit human psychology. Disinformation campaigns may create highly believable false evidence using AI.
To defend their firms, security leaders must update strategies and training. Simulating AI-enhanced threats during exercises helps identify vulnerabilities. Involving ethicists in risk reviews promotes AI aligned with company values.
Here’s an example:
A cybercriminal group uses AI to study a company’s public communications. The AI then generates a phishing email mimicking the CEO’s writing style. This tricks an employee into wiring funds to the criminals.
By training staff to identify AI-generated text, businesses can better spot and stop attacks. Running simulated AI phishing drills also improves responses. Proactive defense minimizes damage.
The Black Box Challenge
The complexity of AI systems like ChatGPT makes them hard to audit thoroughly. This “black box” issue creates governance and compliance challenges.
Regulators want more AI explainability to ensure fairness and prevent discrimination. While full transparency isn’t feasible yet, some solutions exist. Documenting training data sources provides useful context about potential biases. Emerging tools for probing AI decisions are also helping.
Pursuing explainability builds trust despite limited visibility into AI’s inner workings. Ongoing research aims to shed more light on these black box systems.
Here’s an example:
A lending institution uses AI to assess loan applicants. But regulators want to ensure the AI does not make unfair decisions based on race, age or gender.
By documenting exactly what data is used to train the AI, the lender provides assurance no prohibited factors are considered. New algorithms also help probe the AI’s reasoning on specific cases when needed. This supports compliance and accountability.
Reviewing AI-Generated Code
For software teams, AI coding promises huge efficiency gains. However, blindly integrating unvetted code is very risky.
Bugs or vulnerabilities could critically disrupt operations, enable data breaches and corrupt systems. All AI code requires rigorous reviews, testing and approvals pre-launch.
Human oversight is still essential in AI-assisted development. Continuous performance monitoring also catches emerging issues over time. With diligence, AI can significantly boost development speed and quality.
Here’s an example:
A start-up uses AI to accelerate writing new code for its product. But in its haste, it deploys the code without testing it thoroughly.
Soon after launch, bugs crop up that cause severe service outages. Users’ personal data is also exposed publicly due to flaws in the AI-generated code.
Preventative measures like code audits, penetration testing, and gradual rollout could have avoided this. The start-up resolves to implement prudent devops with all future AI coding assisting engineers.
Moving Forward Responsibly
Sustainable success with AI requires focusing on people as much as technology. Companies can adopt AI responsibly by:
- Reviewing risks for all new AI uses, data and integrations
- Making careful policies governing access permissions and oversight
- Ensuring human validation of AI outputs before acting on them
- Maintaining human accountability over all AI systems
- Enabling ways to probe opaque aspects of complex AI tools
- Continuously monitoring AI for evolving performance issues
- Incorporating simulated AI threats into security drills
- Aligning AI with ethical values and social responsibility
With robust precautions, companies can tap into leading-edge AI securely. Oversight ensures tech aligns with human values. If done right, AI can drive new growth while minimizing downsides. The future looks bright for AI and business together!
Key AI Terms
- AI ethics - Ensuring AI operates fairly, safely and aligned with moral values.
- Trustworthy AI - AI systems that are transparent, manage risks responsibly and protect data rights.
- Explainability - Making opaque AI systems more understandable to humans.
- Uncertainty - AI predictions have degrees of uncertainty which should be weighed appropriately.
- Environmental impact - AI’s energy use creates substantial carbon emissions contributing to climate change.
- Cloud computing - Delivering on-demand AI computing power via the internet.
- Intellectual property - Innovations protected by patents, copyrights and trademarks.
- Source code - The programming code that software is built from.
- Trade secrets - Confidential business information providing competitive advantage.
- Customer data - Information collected about customers and their behaviors.
- Private information - Personal data and other sensitive details.
- Confidential information - Sensitive business data meant only for authorized access.
- Contractual obligations - Commitments made between businesses required by agreements.
- Regulatory obligations - Requirements businesses must comply with under laws.
- Plagiarism - Passing off others’ work as your own.
- Deepfakes - AI-generated fake images, videos and audio that seem real.
- Copyright infringement - Using copyright-protected work without permission.
- Safety - Protecting people from harm from AI.
- Reputational damage - Loss of trust and credibility from AI-related incidents.
- Bias - Unfair prejudice in AI reflective of poor training data.
- Discrimination - Unjust treatment based on protected characteristics like race or gender.
Let me know if the simplified explanations help make the key concepts more accessible and understandable for readers. I’m happy to clarify or refine any of the AI terms further.
References
Deloitte. “Trustworthy AI.” 2018.
Metz, Cade. “A.I. Systems Should Be Accountable for Their Actions.” The New York Times, 2022.
Frequently Asked Questions
What is generative AI?
Generative AI refers to advanced systems that can create brand new content like text, images, audio and video. Unlike traditional AI, they don’t just analyze data - they can produce their own original output. Popular examples are ChatGPT, DALL-E 2 and GitHub Copilot.
How can businesses use generative AI?
Common uses include creating marketing copy, answering customer service questions, generating code, analyzing data to find insights, automating workflows and assisting human developers. The technology is rapidly spreading across industries.
What risks does generative AI have for companies?
Major risks include biased or false outputs, cybersecurity vulnerabilities, compliance issues with handling sensitive data, legal liability if AI content causes harm, lack of transparency into how systems work, and integrating unvetted AI-generated code.
How can businesses mitigate generative AI risks?
Strategies include comprehensive risk assessments, strict access controls for data, validating all AI outputs, maintaining human oversight, monitoring systems for issues, aligning models to ethical values, training staff on risks, and having robust review processes for AI code before deployment.
What regulations apply to generative AI?
Current laws related to privacy, cybersecurity, consumer protection, copyright, accessibility, free speech and bias may apply depending on the use case. But regulations specific to AI are still emerging. Organizations should monitor legal obligations in jurisdictions where they operate.
How can I optimize generative AI content for SEO?
Conduct keyword research for topics to target. Produce lots of long-form, high quality content rich in semantic keywords. Include structured data markup. Generate multimedia formats like images, videos and podcasts. Promote across social media, outreach and PR. Analyze search performance to refine over time.
What are some best practices for securing generative AI?
Robust access controls, encryption, data anonymization, model monitoring, output validation, penetration testing AI code, human-in-the-loop review procedures, continuous auditing capabilities, and comprehensive cyber resilience strategies anchored in zero trust principles.
How do companies develop a generative AI policy?
Document acceptable use guidelines based on risk assessments. Outline data access procedures, human oversight requirements, controls, audits, and ethics principles. Maintain accountability with executive approval workflows. Provide staff training on policies and risks. Regularly review and update policies as technology and regulations evolve.
Disclaimer: This article was generated automatically using AI to provide general information about enterprise risks with generative AI and ChatGPT. It should not be considered professional or legal advice. The content does not necessarily represent the views of the publisher. Please consult qualified professionals regarding your specific needs and obligations.